1.1 Fear of the double
Although the understanding of simulations as false or illusory was largely suppressed after World War II, a deep suspicion toward representation has left its mark on both science and art. Literary history abounds with faulty replicas and despicable twins, from the watery reflection of Narcissus to Dr. Jekyll’s murderous alter ego and the seductive usurper in Fyodor Dostoevsky’s The Double. They appear as living specters: likenesses that unearth painful truths for their real-life counterparts leading them to madness, solipsism, and death.
A splintered self is not always a hindrance. In the Tang Dynasty folk tale “The Divided Daughter,” the protagonist defies her parents’ wishes by running off to live in the imperial capital with her lover. Five years later, when feelings of guilt drive her home again, the young woman’s father explains that his daughter has been bedridden all this while and never left the house. The prodigal daughter enters her former bedroom and the two women become one—grateful for everything an indivisible self could never have experienced.1
Such misgivings in Western literature may well be an output of classical Western philosophy, with its tendency to boil over into full-blown existential paranoia. Plato’s “Allegory of the Cave” ought not to have been taken literally but has been faithfully upgraded over the centuries—whether in the shape of René Descartes’s Deus deceptor or the computational simulation of David Chalmers’s David Chalmers’s Reality+: Virtual Worlds and the Problems of Philosophy .
Perhaps a greater understanding of what simulations are and do would decrease the likelihood of future philosophers assuming their imposition from outside. In Beyond Good and Evil (1866) , Friedrich Nietzsche dismissed the theory that an operating system runs beneath our capacity to detect it: “It is, in fact, the worst proved supposition in the world.” Of the simulation hypothesis specifically, Nobel Prize-winning physicist Frank Wilczek soberly asked on Sean Carroll’s Mindscape podcast in January 2021: “If this is a simulated world, what is the thing in which it is simulated made out of?”
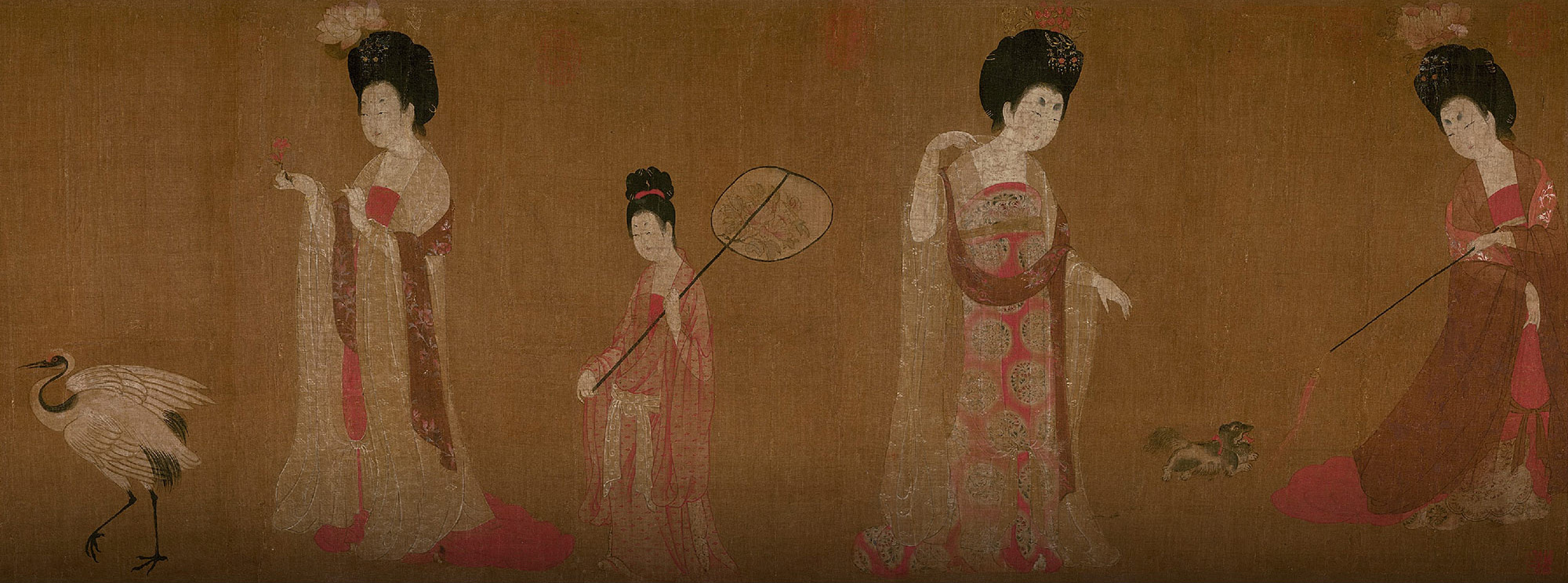
1.2 What are simulations?
Simulations are a tool—a constructive apparatus that is most fruitful in situations where existing knowledge breaks down. More precisely, they are a dynamic reconstruction of a complex process or system which aids the user’s understanding of that system or process. Simulations are not flawed copies of a “more true” reality, but rank among the most effective strategies we have for increasing knowledge about the world. It is the “partitive nature” of simulations, however—the fact that someone must decide what to include and what to ignore—that has led to some anxiety toward them as an epistemic tool.2
The terms model and simulation are often used interchangeably. They are closely linked but are not quite the same. Model, from the Latin modulus, meaning “measure,” refers to an “informative representation of an object, person, or system.”3 Models are “interpreted structures” whose purpose can be folded in with their ontology. It is a common mistake to assume that all models aspire toward verisimilitude, or realistic representation. But by their very nature models must target salient features selected according to the model maker’s purposes.
Simulations are best described as the execution of a single model, or multiple overlapping models, over time. You build a model, but you run a simulation. Both are ways of measuring, but models target the conceptualization or functional abstraction of real-world systems, whereas simulations are more focused on their implementation. A simulation can be disturbed, idealized, or incrementally transformed in order to record the shifting correspondence between it and the system it aims to represent. Noise and randomness can be introduced to create stochastic simulations with little knowledge of what the outcome will be.
Simulations are a crucial interpretative tool for capturing reality in a shared context. In this way they resemble other cognitive framing technologies as outlined in the table below—distinct categories we might be tempted to rank in terms of usefulness. Our instinctive bias as carbon-based intelligences might lead us to privilege in vitro experimentation over in virtuo computer simulations, seeing one as material and the other less obviously so. But they are equivalent, even if different. In fact they are often complementary.
In certain fields, a computer simulation is the only possible approach to discovery, such as when studying the origins of the universe, for example, or unpacking the formation of planetary systems from interstellar dust, neither of which can be recreated in a laboratory.
In Reconstructing Reality (2014) , philosopher Margaret Morrison argues that there is no justification for epistemically privileging the results of experiments over knowledge accessed using idealizations, abstractions, and fictional models. One reason for this is that impossible mathematics can enable new discoveries. An infinite system is essential to explain phase transitions in statistical mechanics, for example. Likewise an infinite population improves results in genetics despite the fact that no such population could exist.
A simpler way to understand the significance of simulations in the modern world is to consider airline pilots. Each pilot is trained in a flight simulator containing software that is far more complex than the code onboard an actual airplane needs to be. Every nine months, pilots return to the airline’s training facilities to ensure their familiarity with the latest procedures, emergency situations, and aircraft systems. These expensive devices are built from physical, mathematical, and computational parts, the three categories into which most simulations can be placed.
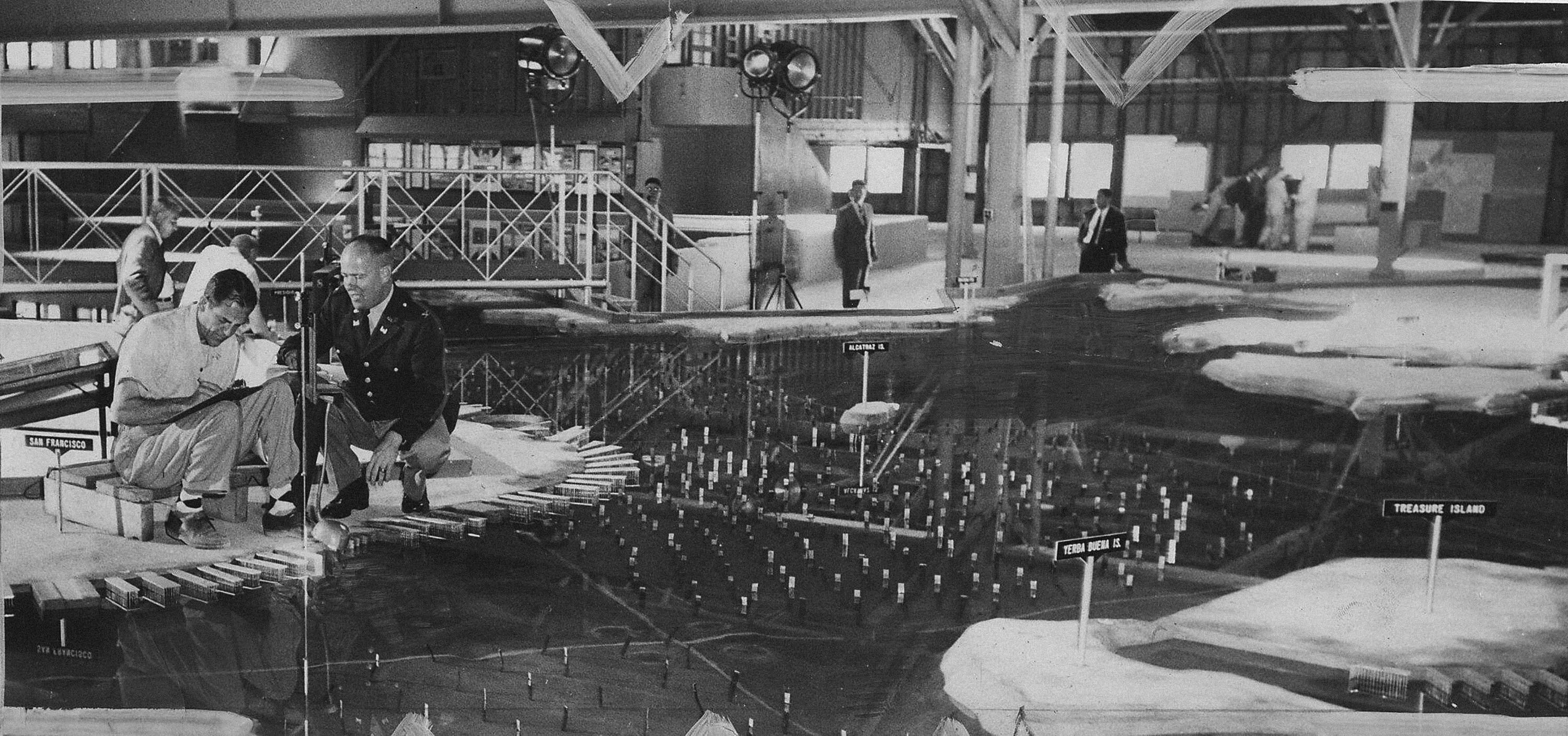
1.3 Three types of model
John Reber was an amateur musical theater producer and schoolteacher in 1950s’ California who was anxious about the Bay Area’s fresh water supply. Despite a lack of expertise, he developed an ambitious plan to dam up the bay. Reber was no city planner, but he was influential and he knew how to generate funds and popular support. In 1957, fearing that the plan might actually be given the go-ahead, the US Army Corps of Engineers built a hydraulic scale model to test Reber’s plan, complete with pumps that simulated fluid dynamics including tides, currents, and even the salinity barrier where fresh and salt waters met. The model was 1:1000 scale horizontally, 1:100 scale vertically, and replicated the twenty-four tidal cycle just under once every fifteen minutes.
Although Reber died before seeing the results, the model indicated that damming up the San Francisco Bay would cause flooding and structural damage, as well as being an enormous waste of resources. The Bay Area Model later became a museum: A remarkable example of a concrete or physical model like the many others that have aided scientific experimentation or determined future projects for centuries.4
The majority of scientific models fall into one of three categories: concrete, mathematical, or computational. There are also edge cases like “model organisms,” which are easy-to-handle analogs where biochemistry, gene regulation, and behavior is regular across the class under study (as in the use of yeast or Escherichia coli in microbiology, or mice as a stand-in for humans in mammal studies). More often than not they conform to one of the three groups.5
Examples of mathematical models might include the Lotka-Volterra model of predator-prey dynamics developed to anticipate how fish stocks in the Adriatic Sea would respond to increased fishing activity following World War I. An example of a well-known computational model is economist Thomas Schelling’s segregation model, which used a 51×51 square grid and a random distribution equilibrium to transform even a mild preference toward in-group neighbors into notable segregation even without economic or policy interventions.
Schelling's model of segregation
Simulations remain closely tied to the purposes for which they were designed. At the same time there are features that commonly recur, such as whether they are stochastic, idealized, or deterministic (i.e., whether randomness, noise, or completeness is intentionally introduced). Specific simulations may be associated with distinct fields, such as Monte Carlo in finance, N-body simulations in astronomy, or reaction diffusion models in biochemistry.
1.4 Simulations and simulationism
Many simulations are used in search of ground truth, many others intentionally divert us from the truth. These other, parallel simulations may take place in virtual or augmented reality, in the form of game worlds or social simulations. They may take advantage of spectacular three-dimensional (3D) environments or the phenomenological superimposition of spatial computing. But there is no technological precondition for creating simulations capable of reshaping how society understands itself.
There are already thousands of cultural models which actively resist any incursion from fundamental or baseline reality. These closed worlds include everything from dictatorial or theocratic regimes to conspiratorial worldviews, or powerful nostalgias projected on the past. Any suite of aesthetic properties, value judgments, and moral suppositions that allow groups to cohere around a shared purpose—be that a nation, worldview, personality, or brand—runs the risk of fixity: a simulation too brittle to update itself.
This is perhaps the paradox at the heart of simulations. For every cosmological model that directs thought toward a deeper understanding of the universe, there is another in the world ready to distract us from it. Crucially, they most likely run on the same computers, processed using algorithms with a shared ancestry. It’s important to ask how parallel technologies can end up with such wildly different relationships to truth, especially in a technological landscape where simulations themselves become sovereign actors—the basis for human, machine, and planetary intelligences, and a core governance mechanism animating factories, designing cities, and deploying geotechnologies.